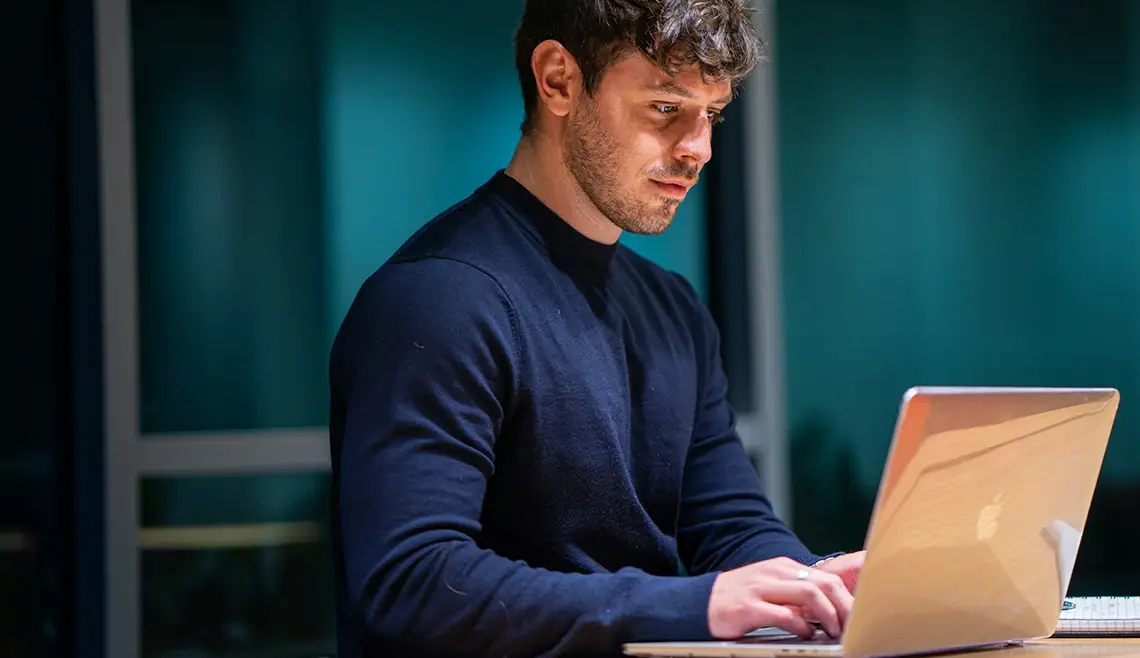
As threats become more complex—with AI-powered cyberattacks—it’s essential that companies adopt advanced approaches to both prevent and respond to incidents. The challenge is that many organizations don’t know where to start. Often, they lack the internal skills to identify concrete use cases or the resources to implement them. But keeping pace with the threat landscape is crucial: falling behind can lead to increased risk to data, service disruptions, and reputational damage.
Meeting regulatory demands
Successful cyberattacks are costly—not just financially, but also in terms of reputation and trust. In regulated environments, organizations must also be able to restore services within specific timeframes to ensure operational continuity. But beyond response, proactive prevention is now a requirement: reacting alone is not enough—you must anticipate. Poor risk management can lead to serious impacts on clients or users, and in many cases, force companies to compensate for damages. That’s why using tools like AI isn’t just a competitive advantage—it’s a necessity to strengthen cybersecurity posture and demonstrate robust control environments.
Threat intelligence and prediction
AI can analyze both global intelligence and company-specific data to identify threats early. Natural Language Processing (NLP) tools can scan sources like the dark web, cybersecurity reports, and industry databases to detect early warning signs. In addition, machine learning algorithms can review historical patterns to anticipate possible attack vectors. Predictive models can assess the likelihood of specific threats—such as ransomware or phishing—based on past data, suspicious behavior, and real-time analysis. These capabilities can even identify unique vulnerabilities in a company’s infrastructure. AI can also assign risk scores and prioritize threats based on their sophistication, impact, and likelihood, helping organizations allocate resources more efficiently.
AI-powered detection and monitoring
One of the most developed use cases for AI in cybersecurity is real-time threat and anomaly detection in networks and systems. Machine learning models can monitor network traffic and reduce the risk of DDoS attacks or data theft. Behavioral analytics can also detect if a user or application is acting unusually, which may indicate a compromise. AI can monitor endpoints (such as laptops or mobile devices), as well as IoT devices, to detect suspicious activity. In the area of privileged access management, behavioral modeling helps identify improper access to sensitive information before real damage is done. These capabilities are increasingly essential. For example, CrowdStrike’s latest Global Threat Report found that 79% of detected attacks did not use malware but relied on compromised credentials. Combined with identity and access management (IAM) systems, these tools can automatically adjust permissions and respond to abnormal behavior in real time.
Automated incident response
When an incident occurs, organizations must act quickly to protect systems and restore services while meeting their operational resilience goals. Although many already have response protocols in place, they often don’t take full advantage of automation. Automated responses include blocking malicious IP addresses, isolating infected devices, or disabling compromised accounts. Additionally, AI-driven decision models can tailor responses based on the incident type and regulatory context. Smart threat containment helps identify affected systems, analyze attack spread, and isolate critical assets without disrupting business operations. Integrations with SOAR (Security Orchestration, Automation and Response) tools can apply predefined remediation protocols and speed up recovery. After an incident, AI can assist in forensic analysis to identify attack vectors and exploited vulnerabilities. NLP tools can even generate automatic reports to meet regulatory requirements and offer preventive recommendations.
Training and awareness with AI
AI can also enhance cybersecurity training by simulating attacks and promoting a stronger risk-aware culture. Phishing remains one of the most common attack vectors, and AI enables customized simulations based on real threats. By combining this with behavioral analytics, it's possible to identify more exposed profiles—such as employees handling sensitive data—and tailor training to their needs. Cybercriminals are already using AI to locate high-value or vulnerable targets (“whales”); organizations can use the same technology to get ahead of those risks, offering interactive and adaptive awareness campaigns.
Continuous learning and adaptation
A solid cybersecurity strategy requires constant refinement. Companies must continue to train their AI models with new data and test them using techniques like adversarial learning, simulating cyberattacks to assess model effectiveness. It’s also critical to learn from every incident, participate in expert forums, and apply regulator recommendations to improve system performance. Let’s not forget AI can also pose risks: there are documented cases of intentionally manipulated (“poisoned”) models with hidden backdoors that attackers can exploit. This turns AI itself into a new attack surface, making it essential to have expert guidance when designing, deploying, and governing these systems securely.
Automating regulatory compliance
AI can help organizations comply with regulations such as the General Data Protection Regulation (GDPR) or data security standards like PCI-DSS. Machine learning algorithms can feed risk management dashboards, detect anomalies in real time, and simplify reporting. Additionally, NLP can identify new regulatory guidelines and automatically adjust compliance controls. Still, like any AI application, clear governance must be in place to oversee and validate these activities.
Where to start?
As AI becomes more relevant, its role in cybersecurity grows. Organizations relying solely on manual processes may fall behind in the face of increasingly automated threats. To get off on the right foot, it's advisable to form a cybersecurity committee with key stakeholders—from leadership to IT, data scientists, and compliance experts—to define AI’s role within the broader strategy. This includes usage policies, data privacy, technical limitations, and alignment with current regulations. As with any risk management approach, it’s crucial to define metrics, establish effective governance processes, and track outcomes. Only then can organizations move toward a resilient, future-ready cybersecurity environment.
At Grant Thornton, we help organizations strengthen their cybersecurity strategy—enhancing their ability to prevent, detect, and respond to ever-evolving threats.